In recent years, the use of Autonomous Underwater Vehicles (AUVs) has significantly expanded for various purposes, such as environmental monitoring, mapping, submarine cable and wreckage inspection, and search-and-rescue operations. This growth is primarily attributed to advancements in on-board computational power and enhanced underwater human-robot interaction capabilities, which enable AUVs to interact effectively with human divers without assistance from a topside operator. Recent research [1] allows AUVs to determine diver attentiveness and plan trajectories for interaction if needed, making them more powerful for collaborative missions with human divers. To ensure effective collaboration, AUVs must be intelligent, understand the surroundings and recognize the current activity of their dive buddies to make informed decisions. For example, during critical tasks, such as rescue operations, it is crucial for the AUV not to disrupt the diver’s focus, allowing them to concentrate entirely on their responsibilities without unnecessary disturbance. Therefore, it is important for an AUV to have the capability to recognize the current activity of a diver.
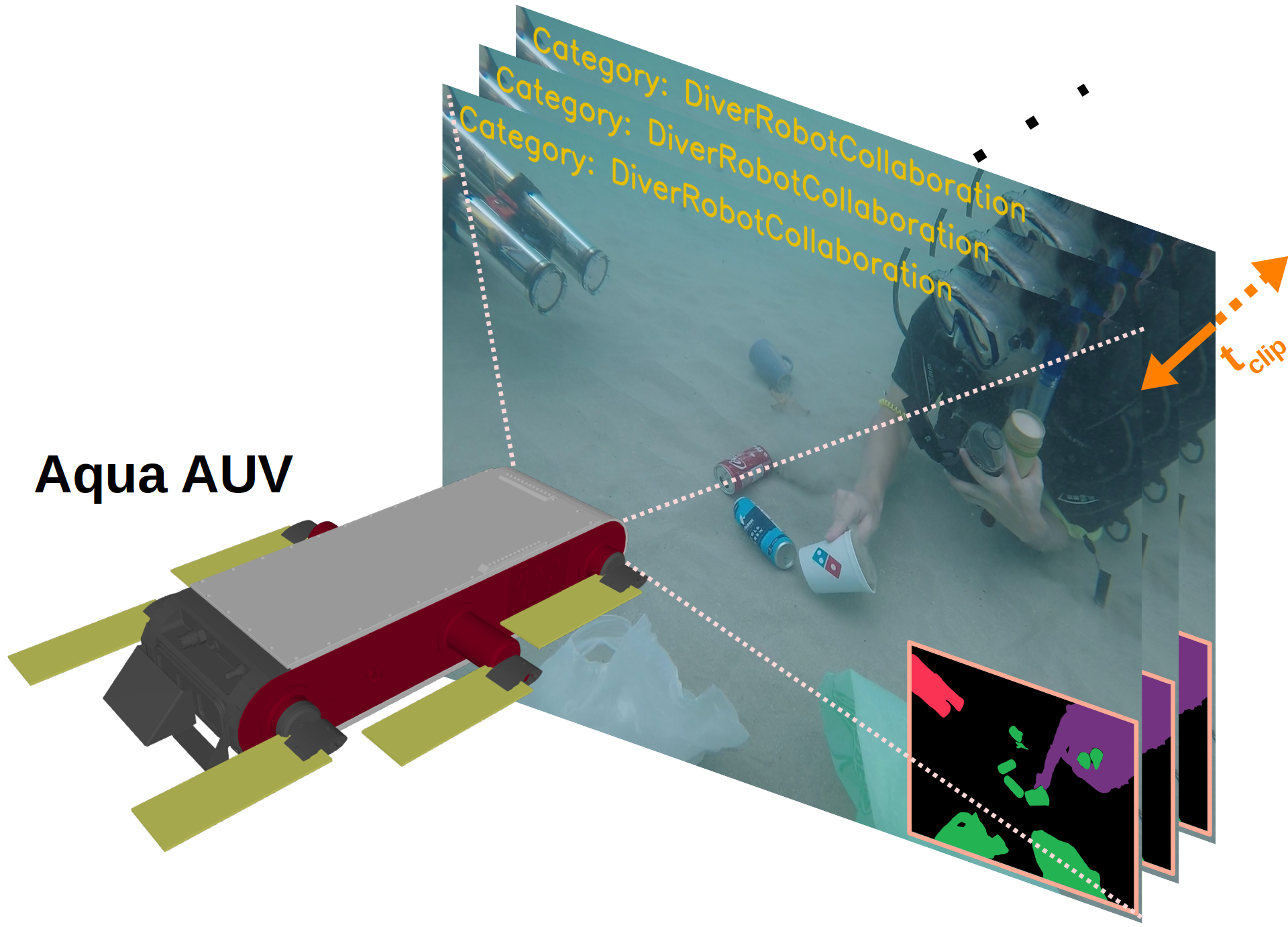
In this work, we present a novel recognition framework to analyze and understand diver activities underwater (see Fig. 1). The main element of the proposed framework is a transformer-based architecture [2], which can learn highly discriminative spatio-temporal features from underwater human-robot collaborative scenes. Recent research (e.g., [3], [4]) indicates that significant performance improvements can be attained by constraining the spatial area of interest that models learn from. To this end, we propose supervising the training of our model with scene semantics to enforce the network to focus on important elements in the scenes that are significant for analyzing the activity of the divers. To enable this, we formulate the first-ever Underwater Diver Activity (UDA) dataset, consisting over 2400 semantically segmented images depicting various activities of divers in a multi-human-robot collaborative setting, with pixel annotations for divers, robots, and objects of interest. We conduct comprehensive experimental evaluations of the proposed framework, showcasing its effectiveness in comparison to various existing state-of-the-art activity recognition models.
Paper:
UDA Dataset: https://drive.google.com/file/d/1mVapKpNWNM8bUro2kssiElckZDdccEtI/view?usp=sharing
References:
[1] S. S. Enan and J. Sattar, “Visual Detection of Diver Attentiveness for Underwater Human-Robot Interaction,” arXiv preprint arXiv:2209.14447, 2022.
[2] S. S. Enan, M. Fulton, and J. Sattar, “Robotic Detection of a Human-Comprehensible Gestural Language for Underwater Multi-Human-Robot Collaboration,” in 2022 IEEE/RSJ International Conference on Intelligent Robots and Systems (IROS), 2022, pp. 3085–3092.
[3] M. J. Islam, P. Luo, and J. Sattar, “Simultaneous Enhancement and Super-Resolution of Underwater Imagery for Improved Visual Perception,” in Robotics: Science and Systems (RSS), 2020.
[4] M. J. Islam, R. Wang, and J. Sattar, “SVAM: Saliency-guided Visual Attention Modeling by Autonomous Underwater Robots,” in Robotics: Science and Systems (RSS), NY, USA, 2022.